Predicting and Improving ADMET Properties of Medications with Machine Learning
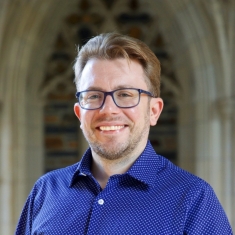
Daniel Reker, PhD
Duke University, Durham, NC
Abstract: In drug discovery and development, understanding a drug's ADMET properties (Absorption, Distribution, Metabolism, Excretion, and Toxicity) is crucial for determining the drug’s safety and efficacy. Machine learning models are increasingly being developed to triage and support ADMET characterization; however, these models suffer from limited data availability. We develop novel machine learning approaches to predict ADMET properties of investigational and approved drugs to streamline characterization and detect potential ADMET liabilities more rapidly and effectively. This involves the design of novel machine learning approaches that can learn from limited data more effectively. Additionally, we couple our algorithms to novel experimental platforms to generate the most useful data for the algorithms. We also use such approaches to examine currently under-investigated drug toxicities, such as the impact on the composition and metabolism of the microbiome. Our machine learning models have predicted several chemotherapeutics that potently inhibit the growth of commensal bacteria and we have validated these effects in microbial culture experiments. We are also exploring how machine learning models can help us circumvent such ADMET challenges for existing drugs by developing innovative drug delivery solutions. For example, we use machine learning to predict excipient-drug interactions that can alter the ADMET properties of a drug. Overall, our laboratory aims to use machine learning to improve drug development and delivery with the goal of developing safer and more effective treatments for every patient.
Biography: Daniel Reker is a tenure-track Assistant Professor in the Department of Biomedical Engineering at Duke University and a Duke Science and Technology Fellow. He holds a B.Sc. with honors in Computer Science and a M.Sc. with honors in Computational Biology and Bioinformatics. He received his PhD from the Institute of Pharmaceutical Sciences at ETH Zurich and conducted his postdoctoral work in drug delivery as a SNSF Postdoctoral Fellow with Bob Langer and Giovanni Traverso at MIT. Daniel has been named Forbes 30 under 30 in Science and Healthcare and has recently been recognized both as a National Institute of Biomedical Imaging and Bioengineering “Trailblazer” and as a National Institute of General Medical Sciences “Outstanding Investigator”. At Duke, he is building an integrated experimental and computational laboratory to conduct active machine learning campaigns with applications in drug discovery and drug delivery.