Opportunities for Explainable Machine Learning in Mechanical and Aerospace Engineering
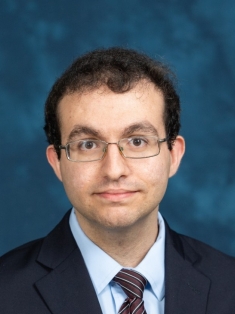
Yossi Cohen, PhD
Department of Mechanical and Aerospace Engineering
Rutgers University-New Brunswick
Abstract:
In recent years, machine learning has become popular for obtaining predictions from high-dimensional datasets in mechanical and aerospace engineering. These data-driven algorithms have enabled key performance indicator improvements such as reducing machine downtime, improving quality, yield, and sustainability. However, as advanced data analytics and digital twin platforms mature in the age of generative AI, there is an unmet need to critically evaluate model outputs to gauge trustworthiness. This presentation will focus on recent efforts in advancing explainable anomaly detection, fault diagnosis, and prognosis of high-dimensional applications in semiconductor manufacturing, aerospace engineering, and renewable power systems. Furthermore, this seminar will describe a new framework for adopting explainable AI in industrial settings under two realistic constraints: a) privacy-encoded inputs and b) weakly labeled or entirely unlabeled datasets. The framework also encompasses an approach for semi-supervised clustering based on Shapley value analysis, with the added capability of accurately describing clusters with measurable inputs. Finally, this seminar will present a vision for future opportunities in engineering enabled by integrating uncertainty quantification and explainable AI techniques for augmented and trustworthy artificial intelligence.
Biography:
Joseph (Yossi) Cohen is an Assistant Professor in the Department of Mechanical and Aerospace Engineering at Rutgers University. He received his Bachelor’s, Master’s, and Ph.D. degrees in Mechanical Engineering from the University of Michigan. His research interests include human-centered augmented intelligence, responsible artificial intelligence in industry, and sustainable manufacturing. Before joining Rutgers, he was a Schmidt AI in Science Postdoctoral Research Fellow at the Michigan Institute for Data and AI in Society, where he investigated the intersection of explainable artificial intelligence with uncertainty quantification to improve the reliability of model explanation methods. At Rutgers, his Trustworthy, Robust, and Understandable SysTems in Mechanical Engineering (TRUST-ME) Lab aims to construct responsible and human-centered methodologies for intelligent optimization of systems and operations to improve decision-making in industry.