Coordination, Optimization and Learning in Multi-Agent Networks (Virtual Only)
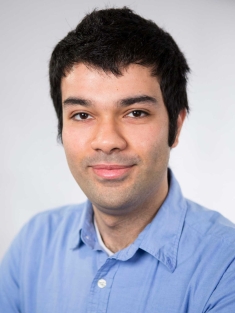
Dr. Shahin Shahrampour
Northeastern University
Zoom Link: https://rutgers.zoom.us/j/93732405973?pwd=THFtWGhwSEZ6VWxIaXUydGdSeUtudz09
Abstract: Complex networks are ubiquitous, with applications in robotics, economics, social science, and smart grids.This talk centers around two fundamental problems in multi-agent networks. In the first part of the talk, we focus on the consensus problem on the Stiefel manifold. While consensus and coordination are well-understood in Euclidean space, ensuring consensus in non-convex environments poses many challenges. We identify conditions under which the consensus dynamics converge to a global optimum on the Stiefel manifold and further show that if agents are close enough to a consensus state, the convergence is exponentially fast and (asymptotically) on par with the rate obtained in Euclidean space. The analysis of this work opens avenues for the investigation of various distributed optimization methods on the Stiefel manifold. In the second part, we discuss multi-agent reinforcement learning (MARL) in Markov potential games. We revisit the independent natural policy gradient (NPG) algorithm and present novel sample complexity results for the case in which an oracle provides exact policy evaluations. Our rate improves upon the previous best-known sample complexity of NPG in MARL and is of the same order as that achievable for the single-agent case.
Biography: Dr. Shahin Shahrampour is an Assistant Professor in the Department of Mechanical and Industrial Engineering at Northeastern University. His research interests include machine learning, optimization, control, distributed and sequential learning, with a focus on developing computationally efficient algorithms for data analytics. Dr. Shahrampour earned his Ph.D. in Electrical and Systems Engineering from UPenn and holds an A.M. in Statistics from Wharton. He was also a postdoctoral fellow at Harvard John A. Paulson School of Engineering and Applied Sciences. Dr. Shahrampour’s research has been recognized by several awards from the National Science Foundation (NSF) to study real-time and distributed learning/optimization and a Best Paper Award at ICASSP 2022 Conference.
Attendance is mandatory for in-person seminar students. For online and part-time students, seminar will be recorded and made available through Canvas.